The results show that the algorithms are at least on par with the traditional prognostic factors. In the near future, it will undoubtedly be possible to have a predictive digital signature of melanoma recurrence.
— Frédéric Staroz, President of the Conseil National Professionnel des Pathologistes (CNPath)
Why
In 2020, over 325,000 people were diagnosed with skin melanoma, with 57,000 deaths in the same year.1 Melanomas represent 10% of all skin cancers and are the most dangerous due to high likelihood of metastasizing (spreading).2
Patients are initially diagnosed with melanoma after a pathologist examines a portion of the cancerous tissue under a microscope. At this stage, the pathologist assesses the risk of relapse—a return of cancerous cells after the melanoma has been treated—based on information such as the thickness of the tumor and the presence of an ulceration.
Combined with factors such as age, sex, and medical history of the patient, these microscopic observations can help a dermatologist assess the severity of the disease and determine appropriate surgical and medical treatment. Preventative treatments can be administered to patients with high likelihood for relapse. However, these are costly and expose patients to significant drug toxicity.
The Solution
Assessing the risk of relapse is a vital but difficult task. It requires specialized training and careful examination of microscopic tissue. Currently, machine learning approaches can help analyze whole slide images (WSIs) for basic tasks like measuring area. However, computer vision has also shown some potential in classifying tumor subtypes, and in time may serve as a powerful tool to aid pathologists in making the most accurate diagnosis and prognosis. 3, 4, 5
The goal in this challenge was to predict whether a relapse will occur in the 5 years following the initial diagnosis using digitized versions of microscopic slides and tabular clinical features. For each slide, participants had to predict a score between 0 and 1 indicating the likelihood of relapse.
The Results
Challenge participants generated over 600 submissions, and the winning solutions achieved log loss scores of 0.39-0.40 (lower is better) compared to 0.50 for a benchmark model that predicted relapse from tabular clinical features. In terms of area under the ROC curve, winning submissions substantially outperformed the benchmark with AUC scores surpassing 0.80.
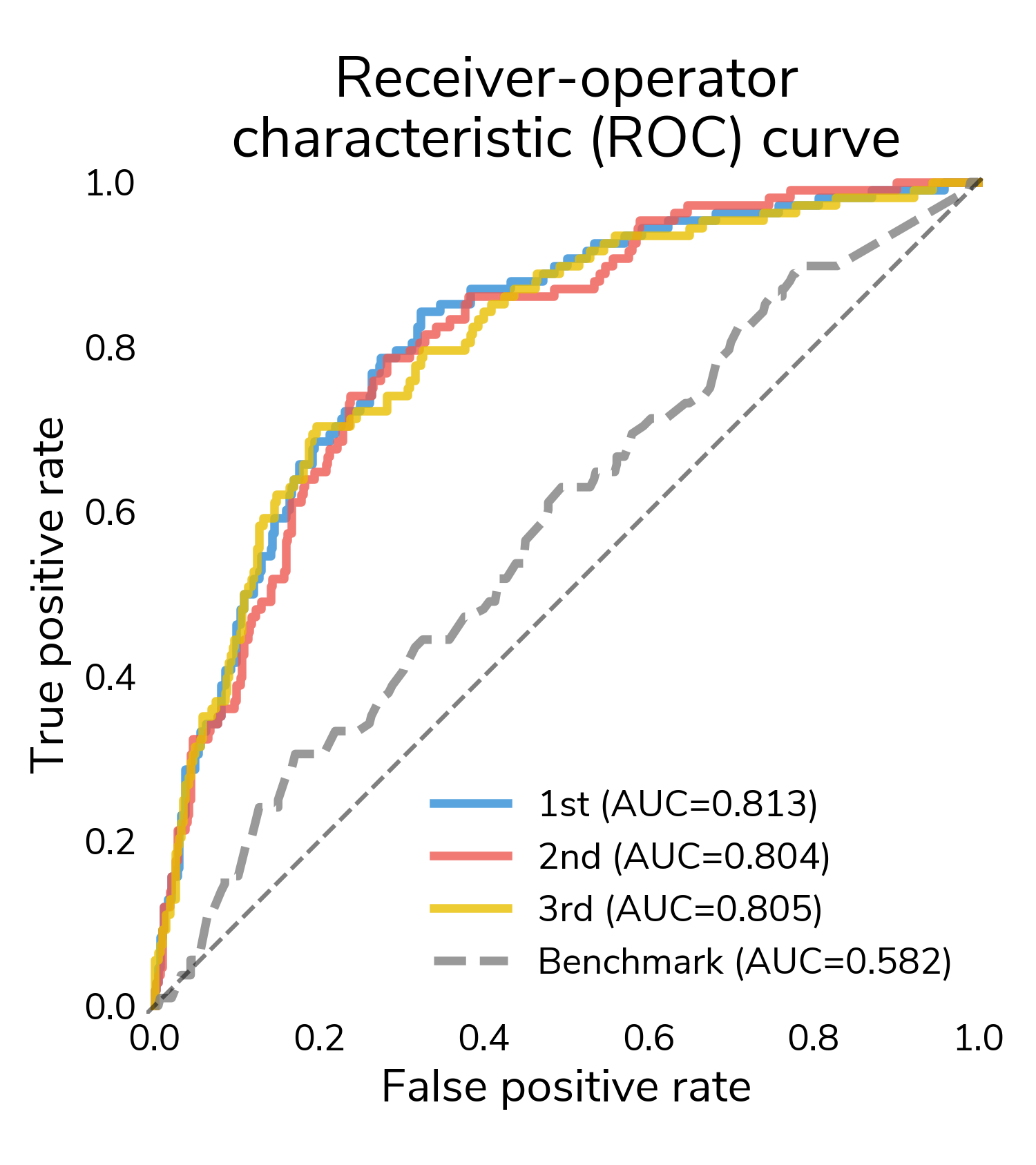
The results suggest that computer vision can detect signals in whole slide images that help make more accurate predictions of relapse months to years into the future.
See the results announcement for more information on the winning approaches. All of the prize-winning solutions from this competition are linked below and available for anyone to continue to use and learn from.
On this page
VisioMel Challenge: Predicting Melanoma Relapse: Rules and Terms of Data Use
DrivenData Competition Rules
GUIDELINES
One account per participant
You cannot sign up to DrivenData from multiple accounts and therefore you cannot submit from multiple accounts.
Private sharing of code
Privately sharing code or data outside of teams is not permitted.
Winner License Type: Open Source License
Winning solutions need to be made available under The MIT License (an open source software license commonly described at http://opensource.org/licenses/MIT) in order to be eligible for recognition and prize money if offered.
Documentation for winning solutions
Winning solutions must be documented using the Winning Model Documentation Template provided to top-ranking participants in order to be eligible for recognition and prize money if offered.
Submission limits
The number of submissions per day is restricted to a fixed value on a per-competition basis. Any attempt to circumvent this limit will result in disqualification.
External data
External data is permitted as long as it is freely and publicly available to all Participants under a permissive open source license (e.g., CC0 or CC-BY) and complies with any external data restrictions stated on the Competition Website.
FULL RULES
NO PURCHASE NECESSARY TO ENTER OR WIN THIS COMPETITION. A PURCHASE OR PAYMENT WILL NOT INCREASE YOUR CHANCES OF WINNING.
BY ENTERING THIS COMPETITION, PARTICIPANT AGREES TO THESE COMPETITION RULES, WHICH ARE A CONTRACT, SO READ THEM CAREFULLY BEFORE ENTERING.
These are the complete, official rules (the "Competition Rules") for the competition (the "Competition") described on the website listed above or linking to these Competition Rules (the "Competition Website"). The Competition Rules incorporate by reference the contents of the Competition Website listed above.
By downloading a dataset linked from the Competition Website, submitting an entry to this Competition (a "Submission"), or joining a Team in this Competition, you are agreeing to be bound by these Competition Rules which constitute a binding agreement between you and DrivenData and, if applicable, any rules and restrictions that may be imposed by the third-party sponsor of this competition (the "Competition Sponsor").
The Competition is hosted by DrivenData, Inc ("DrivenData"). The Competition will run according to the dates listed on the Competition Website (the "Competition Period").
Each registered individual or Team is referred to as a Participant. You may only compete using a single, unique DrivenData account registered at http://www.drivendata.org. Competing using more than one DrivenData account per individual is a breach of these Competition Rules and DrivenData reserves the right to disqualify any individual (or Team including an individual) who is found to breach these Competition Rules.
Eligibility
The Competition is open to all natural persons who are a legal resident of a country, state, province or territory within the Territory, are not the target of any trade sanctions administered or enforced by the United States, and, at the date of entry, are at least eighteen years of age and the age of majority in their jurisdiction of residence. Legal entities and organizations are not eligible for entry. There is no purchase or donation necessary to enter the Competition.
Officers, directors, employees and advisory board members (and their immediate families and members of the same household) of the Competition Sponsor, DrivenData and their respective affiliates, subsidiaries, contractors, agents, judges and advertising and promotion agencies and any family member or member of the same household (whether or not related) of any such persons are not eligible to win a prize in this Competition. For purposes of this Competition, the term “family member” is defined as any spouse, partner, parent, legal guardian, child, sibling, grandparent, grandchild, or in-law.
For the purposes of this Competition, the “Territory” means any country, state, province, territory, region, or jurisdiction where the laws of the United States or local law do not prohibit participating or receiving a prize in the Competition and excludes any area or country designated by the United States Treasury’s Office of Foreign Assets Control. Participants located in the Russian Federation are not eligible to win a prize in this Competition.
Registration
If you meet the eligibility requirements and would like to participate, then you must first complete the Competition registration process through the Competition Website within the Competition Period. After you complete the registration process, you will receive access to the available Competition Dataset(s) (each a “Dataset”) (described on the Competition Website) that will enable you to develop and submit one or more Submissions. All Submissions must be received during the Competition Period. To register, visit the Competition Website and follow the onscreen instructions to complete and submit your registration. All of the registration information that you provide is collectively referred to as your "Account". (If you have already created an Account at
After you register individually, you may join a group of individuals with which to collaborate (each group, a "Team"), but you may register only once for the Competition either as an Individual or as part of a Team. If you register for the Competition more than once, you will be, and the remainder of your Team may be, disqualified in DrivenData’s sole discretion. An individual Participant cannot join more than one (1) Team and a Participant who is part of a Team cannot also enter the Competition on an individual basis.
You acknowledge and agree that you are solely responsible for abiding by your employer's policies regarding participation in the Competition. Participants (including each member of a Team) must not be under any legal or contractual obligation that would prohibit their participation in this Competition as described in these Competition Rules. If participating in this Competition would result in a violation by Participant of any law applicable to them or any agreement to which they are a party or employer or company policies and procedures, such Participant is ineligible. Participants and Teams (including each member of a Team) represent and warrant that their participation in this Competition does not violate their employer’s or company’s policies and procedures; and if participating in this Competition would result in a violation by Participant of employer or company policies and procedures, such Participant is ineligible. If you are participating within the scope of your employment, as an employee, contractor, or agent of a legal entity, you warrant that such party has full knowledge of your actions and has consented thereto, including your potential receipt of a prize.
Once you have completed the registration process, you or your Team will be provided with access to the Dataset(s) that you use to develop your Submissions.
BY ENTERING THE COMPETITION YOU ACCEPT THE CONDITIONS STATED IN THESE COMPETITION RULES INCLUDING THE RULES ON THE COMPETITION WEBSITE, AGREE TO BE BOUND BY THE DECISIONS OF THE JUDGES, COMPETITION SPONSOR AND DRIVENDATA AND WARRANT THAT YOU ARE ELIGIBLE TO PARTICIPATE IN THE COMPETITION. IF YOU DO NOT ACCEPT ALL OF THESE COMPETITION RULES, THEN PLEASE DO NOT REGISTER FOR THE COMPETITION. WE RECOMMEND THAT YOU PRINT OUT A COPY OF THESE COMPETITION RULES FOR YOUR FUTURE REFERENCE.
Teams
FORMING A TEAM. Multiple individuals may collaborate as a Team. You may not participate on more than one Team. Each Team member must be a single individual operating a separate DrivenData account. You must register individually for the Competition before joining a Team. You must confirm your Team membership to make it official by responding to the Team notification message which will be sent to your Account.
TEAM PRIZES. If a Team wins a monetary Prize, DrivenData will allocate the Prize money in even shares between the Team members unless the Team unanimously contacts DrivenData before the Prize is awarded to request an alternative prize distribution.
Entry submissions
Each Submission must be uploaded to the Competition Website in the manner and format specified on the Competition Website.
Unless otherwise specified on the Competition Website, for the purposes of quantitative evaluation of Submissions, Participants agree to process each test data sample independently without the use of information from other cases in the test set. By default, this precludes using information gathered across multiple test samples during training, for instance through pseudo labeling. Eligible Submissions and models must be able to run inference on new test data automatically, without retraining the model.
All Submissions must be received during the Competition Period. The Submission includes all data, content, copy and/or all other materials submitted in the manner and format specified on the Competition Website via the submission form, as well as all documentation provided by entrants for winning Submissions. The number of Submissions a Participant may submit during each calendar day of the Competition Period will be displayed on the Competition Website. If the Competition includes selection of Submissions to be considered for final evaluation and winner determination, the number of Submissions allowed for selection will be displayed on the Competition Website. If the Competition is a multi-stage Competition with temporally separate training data and/or leaderboard data, one or more valid Submissions must be submitted and selected during each stage of the Competition in the manner described on the Competition Website.
USER SUBMISSIONS MUST IN THEIR ENTIRETY COMPLY WITH ALL APPLICABLE FEDERAL, STATE, LOCAL, AND INTERNATIONAL LAWS AND REGULATIONS. WITHOUT LIMITING THE FOREGOING, IN ORDER TO BE ELIGIBLE, YOU WARRANT THAT YOUR SUBMISSION FOR THIS COMPETITION MEETS THE FOLLOWING SUBMISSION CRITERIA, ALL AS DETERMINED IN THE SOLE DISCRETION OF COMPETITION SPONSOR AND/OR DRIVENDATA:
- Does not include or anticipate the inclusion of any content that is in violation of or infringes third party intellectual property rights including, but not limited to copyrights, including music copyrights, patents, trade secrets, trademarks, and rights of publicity or privacy.
- Is free and clear of all liens, claims, encumbrances or demands of any third parties.
- Does not include or anticipate the inclusion of any unsuitable or offensive content, including nudity, sexually explicit, disparaging, libelous or other inappropriate content.
- Has not been entered in previous challenges, competitions or contests, or won previous awards.
- Has not been published or distributed previously in any media.
- Is suitable for a general audience.
- Does not contain any claims that are not and cannot be substantiated or that would be false and/or misleading to a reasonable consumer.
Selection of winners
This Competition is a challenge of skill and the final results are determined by evaluating a combination of quantitative and qualitative factors, as more fully described on the Competition Website. For that portion of the Competition evaluated quantitatively, the results will be determined solely by leaderboard ranking on the private leaderboard based on an objective statistical evaluation metric published on the Competition Website prior to commencement of the Competition (subject to compliance with these Competition Rules). Participants' scores and ranks on the Competition Website at any given stage of the Competition will be based on the objective statistical evaluation metric described on the Competition Website, as determined by applying the predictions in the Submission to the ground truth of a validation dataset whose instances were a fixed set sampled from the Data. Scores displayed on the public leaderboard while the competition is running may or may not be the same as the final scores on the private leaderboard, depending on how samples from the Data are used for evaluation. For that portion of the Competition evaluated qualitatively, the results will be determined by a panel of judges, as more fully described on the Competition Website. As more fully described on the Competition Website, the Competition may have a quantitative winner, a qualitative winner, a combined quantitative and qualitative winner, or all three.
The evaluation metric used for scoring and ranking Submissions will be displayed on the Competition Website.
Any prize awards are subject to verification of eligibility and compliance with these Competition Rules. All decisions of DrivenData, the Competition Sponsor and/or judges will be final and binding on all matters relating to this Competition. DrivenData reserves the right to examine the Submission and any associated code or documentation for compliance with these Competition Rules. In the event that the Submission demonstrates a breach of these Competition Rules, Competition Sponsor or DrivenData may at its discretion take either of the following actions:
- disqualify your Submission(s); or
- require that you remediate within one week any issues identified in your Submission(s) (including, without limitation, the resolution of license conflicts and confirmation of the fulfillment of all obligations required by software licenses). DrivenData is under no obligation to alert you of any issues with your Submission or permit you to remediate any identified issues.
A tie between two or more valid and identically ranked submissions will be resolved in favor of the tied submission that was submitted first.
A Participant may decline to be nominated as a Winner by notifying DrivenData directly within one week following the Competition deadline, in which case the declining Participant forgoes any prize or other features associated with winning the Competition. DrivenData reserves the right to disqualify a Participant who so declines at DrivenData's sole discretion if DrivenData deems disqualification appropriate.
Prizes and conditions
If the Competition has a prize, the Prize Winner(s) will be notified within 10 days of the end of the Competition on the Competition Website. The Prize Winner(s) will receive their prizes awarded as a check or electronic transfer from DrivenData.
If a Team wins a prize, all Team members must submit a single written statement describing how the prize is to be allocated among the Team members. If the Team fails to submit such statement within 10 days after DrivenData requests it, then DrivenData will distribute the prize among Team members in equal shares and will have no further obligation to winning Team members.
As a condition of receipt of a prize, winner must deliver their solution’s code and documentation to DrivenData. The source code must contain a description of resources required to build and run the solution, and should be able to sufficiently reproduce winning results and generate predictions on new data samples with and without retraining. The accompanying documentation should be consistent with DrivenData’s Winning Model Documentation Template, which will be provided to winners after the competition.
The prize may be awarded to an alternate winner if required documentation is not returned within fifteen (15) days after mailing to winner, if prize notification letter/email or prize is returned as undeliverable, if winner does not respond to an email or other communication from DrivenData within seven (7) days of the date sent, or if the winner, in DrivenData’s judgment, does not respond to reasonable requests for information related to prize delivery or is otherwise unable or unwilling to coordinate prize delivery within fifteen (15) days after prize notification. Allow thirty (30) days for prize delivery. By accepting any prize/award, Winner agrees to allow DrivenData and/or the Competition Sponsor to disclose Winner’s first name, first initial of last name, and city of residence, as well as prize information to the extent required by law, and if so required by law, Winner agrees to the disclosure of additional personal information, all without additional compensation.
DrivenData is responsible only for prize delivery and is not responsible for prize utility or otherwise. No substitution or transfer of prizes is permitted. All taxes, fees and expenses associated with participation in the Competition or receipt and use of a prize are the sole responsibility of the Prize Winner(s).
PARTICIPANT INTELLECTUAL PROPERTY LICENSING/ASSIGNMENT. All Participants grant DrivenData, the Competition Sponsor and/or judges a limited, worldwide license to use each Participant’s Submission for the administration of the Competition.
As a further condition of receipt of a Prize, each winning Participant thereby licenses their winning Submission and the source code used to generate the Submission according to the Winner License/Assignment Type specified above (note: if no Winner License/Assignment Type is specified above, the Winner License/Assignment Type is deemed to be Non-Exclusive License).
NON-EXCLUSIVE LICENSE. If the Winner License/Assignment Type for the Competition (see Winner License/Assignment Type above) is a Non-Exclusive License then each Winner by accepting a Prize thereby:
- grants to Competition Sponsor and its designees a worldwide, non-exclusive, sub-licensable, transferable, fully paid-up, royalty-free, perpetual, irrevocable right to use, not use, reproduce, distribute, create derivative works of, publicly perform, publicly display, digitally perform, make, have made, sell, offer for sale and import their winning Submission and the source code used to generate the Submission, in any media now known or hereafter developed, for any purpose whatsoever, commercial or otherwise, without further approval by or payment to Participant; and
- represents that he/she/it has the unrestricted right to grant that license.
OPEN SOURCE LICENSE. If the Winner License/Assignment Type for the Competition (see Winner License/Assignment Type above) is Open Source License, then each Winner by accepting a Prize thereby:
- licenses their winning Submission and the source code used to generate the Submission under the MIT License (an open source software license commonly described at http://opensource.org/licenses/MIT); and
- represents that he/she/it has the unrestricted right to grant that license.
ASSIGNMENT TO COMPETITION SPONSOR. If the Winner License/Assignment Type for the Competition (see Winner License/Assignment Type above) is Assignment to Competition Sponsor, then each Winner by accepting a Prize thereby:
- grants all right, title, and interest in and to their winning Submission and the source code used to generate the Submission, and all intellectual property rights therein, to the Competition Sponsor or their designee;
- represents that he/she/it has the unrestricted right to make that assignment; and
- agrees to execute a stand-alone assignment document in the discretion of the Competition Sponsor or their designee to memorialize the same.
CHEATING. Participating using more than one DrivenData account is deemed cheating and, if discovered, will result in disqualification from the Competition and any other affected Competitions and may result in banning or deactivation of affected DrivenData accounts.
DrivenData reserves the right to request information associated with our investigation of suspected cheating. Failure to respond to these requests (including failure to furnish the requested information) within ten (10) days is grounds for disqualification.
RECEIVING PRIZES. After verification of eligibility, if prizes are awarded, each Prize winner will receive the prize in the form of a check or electronic transfer from DrivenData made out to the Prize winner (if an individual, or to the individual Team members if a Team). Allow 30 days from final verification and confirmation for Prize delivery. Winners may receive an IRS 1099 form or W-8BEN, as applicable, from DrivenData in the amount of their prize at the appropriate time. Prize winners are responsible for any taxes, fees or other liability resulting from their receipt of a Prize.
Permissions
Except where prohibited by law, entry constitutes permission to use winners’ names, hometowns, images, statements and likenesses for online posting, and/or any advertising and publicity without additional compensation. Winners may also be required to sign and return a release of liability, declaration of eligibility and, where lawful, a publicity consent agreement, as conditions of receiving a prize. Failure to comply with the aforementioned conditions shall be grounds for forfeiture of a prize.
Data use and code sharing
DATA. "Data" means the data or datasets linked from the Competition Website for the purpose of use by Participants in the Competition. For the avoidance of doubt, Data is deemed for the purpose of these Competition Rules to include any prototype or executable code provided to Participants by DrivenData or via the Competition Website. Participants must use the Data only as permitted by these Competition Rules and any associated data use rules specified on the Competition Website.
Unless otherwise permitted by the terms of the Competition Website, Participants must use the Data solely for the purpose and duration of the Competition, including but not limited to reading and learning from the Data, analyzing the Data, modifying the Data and generally preparing your Submission and any underlying models and participating in forum discussions on the Competition Website. Participants agree to use suitable measures to prevent persons who have not formally agreed to these Competition Rules from gaining access to the Data and agree not to transmit, duplicate, publish, redistribute or otherwise provide or make available the Data to any party not participating in the Competition. Participants agree to notify DrivenData immediately upon learning of any possible unauthorized transmission or unauthorized access of the Data and agree to work with DrivenData to rectify any unauthorized transmission. Participants agree that participation in the Competition shall not be construed as having or being granted a license (expressly, by implication, estoppel, or otherwise) under, or any right of ownership in, any of the Data.
EXTERNAL DATA. Participants may use external data other than the Data, including pre-trained models, to develop and test their models and Submissions, provided that:
- they comply with any external data restrictions stated on the Competition Website;
- the external data is freely and publicly available to all Participants under a permissive open source license that does not prohibit commercial use (e.g., CC0 or CC-BY).
Furthermore, unless otherwise expressly stated on the Competition Website:
- Participants may annotate provided training data as long as they are included with solutions to enable reproduction and do not overfit to the test set;
- Participants may not add any manual annotations to the provided test data. Eligible solutions need to be able to run on test samples automatically using the test data as provided.
DrivenData and Competition Sponsor reserve the right in their sole discretion to disqualify any Participant who they discover has undertaken or attempted to undertake the use of data other than as permitted according to the Competition Website and in these Competition Rules, in the course of the Competition.
CODE SHARING. Participants are prohibited from privately sharing source or executable code developed in connection with or based upon the Data, and any such sharing is a breach of these Competition Rules and may result in disqualification.
Participants are permitted to publicly share source or executable code developed in connection with or based upon the Data, or otherwise relevant to the Competition, provided that such sharing does not violate the intellectual property rights of any third party. By so sharing, the sharing Participant is thereby deemed to have licensed the shared code under the MIT License (an open source software license commonly described at http://opensource.org/licenses/MIT).
OPEN-SOURCE CODE. A Submission will be ineligible to win a prize if it was developed using code containing or depending on software licensed under an open source license:
- other than an Open Source Initiative-approved license (see http://opensource.org/licenses); or
- an open source license that prohibits commercial use.
Participant warranties and obligations
By registering, you agree that (a) your Account is complete, correct and accurate and (b) your registration may be rejected or terminated and all Entries submitted by you and/or your Team may be disqualified if any of the information in your Account is (or Competition Sponsor of DrivenData has reasonable grounds to believe it is) incomplete, incorrect or inaccurate. You are solely responsible for your Account. All registration information is deemed collected in the United States.
Participation is subject to all applicable federal, state, provincial, territorial and local laws, rules and regulations. You agree to comply with all applicable laws and regulations at all times during your participation in the Competition. Void where prohibited or restricted by law. You are responsible for checking applicable laws and regulations in your jurisdiction before participating in the Competition to make sure that your participation is legal. You are responsible for all taxes and reporting related to any award that you may receive as part of the Competition. You are responsible for abiding by your employer's policies regarding participation in the Competition. Competition Sponsor and DrivenData disclaim any and all liability or responsibility for disputes arising between you and your employer related to this Competition Each Participant must comply with all applicable explicit or implicit terms and conditions or policies applicable for platforms, applications, or software used in connection with or on which their Solution operates. As such, each Participant must follow the spirit of such terms and conditions and policies; and any attempt to subvert or to use a work-around of such terms and conditions or policies may, in DrivenData’s sole discretion, lead to such Participant’s disqualification.
Each Participant is solely responsible for all equipment, including but not necessarily limited to a computer and internet connection necessary to access the Competition Website and to develop and upload any Submission, and any telephone, data, hosting or other service fees associated with such access, as well as all costs incurred by or behalf of the Entrant in participating in the Competition.
By entering a Submission, you represent and warrant that all information you enter on the Competition Website is true and complete to the best of your knowledge, that you have the right and authority to make the Submission (including any underlying code and model) on your own behalf or on behalf of the persons and entities that you specify within the Submission, and that your Submission:
- complies with all applicable federal, state, provincial, territorial, local and international laws, statutes, ordinances, rules and regulations;
- is your own original work, or is used by permission, in which case full and proper credit and identity is given and the third party contributions are clearly identified within your Submission;
- does not contain confidential information or trade secrets and is not the subject of a registered patent or pending patent application;
- does not violate or infringe upon the patent rights, industrial design rights, copyrights, trademarks, rights of privacy, publicity or other intellectual property or other rights of any person or entity;
- does not contain malicious code, such as viruses, timebombs, cancelbots, worms, Trojan horses or other potentially harmful programs or other material or information;
- does not and will not violate any applicable law, statute, ordinance, rule or regulation;
- does not trigger any reporting or royalty obligation to any third party; and
- was not previously published and has not won any other prize/award.
A breach of any of these warranties will result in the corresponding Submission being invalid.
Confidentiality
Confidential Information Defined. As used in this Section, "Confidential Information" means Competition Sponsor materials, and any information provided by DrivenData hereunder, whether of a technical, business, or other nature (including, without limitation, information relating to a party's technology, software, products, services, designs, methodologies, business plans, finances, marketing plans, Sponsors, prospects, or other affairs), that is received by Participant.
Exclusions. Participant's obligations under this Section as to the disclosing party's Confidential Information does not include any information that Participant can document: (a) was known to Participant prior to receiving the same from the disclosing party in connection with this Agreement; (b) is independently developed by Participant without use of or reference to the Confidential Information of the disclosing party; (c) is acquired by Participant from another source without restriction as to use or disclosure; or (d) is or becomes part of the public domain through no fault or action of Participant.
Nondisclosure. In consideration of the terms and conditions of this Agreement, and for other good and valuable consideration, the receipt and sufficiency of which is acknowledged, during and after the term of this Agreement Participant will: (a) use the disclosing party's Confidential Information solely for the purpose for which it is provided; (b) not disclose the disclosing party's Confidential Information to a third party unless the third party must access the Confidential Information to perform in accordance with this Agreement and the third party has executed a written agreement that contains terms that are substantially similar to the terms contained in this Section; and (c) maintain the secrecy of, and protect from unauthorized use and disclosure, the disclosing party's Confidential Information to the same extent (but using no less than a reasonable degree of care) that Participant protects its own Confidential Information of a similar nature. If Participant is required by law or by any governmental or semi-governmental agency or court to disclose the disclosing party's Confidential Information or the terms of this Agreement Participant must give prompt written notice of such requirement to the disclosing party before such disclosure and assist the disclosing party in attempting to obtain an order protecting the Confidential Information from public disclosure.
Return of Information. Upon request, Participant will destroy or deliver to the disclosing party all Confidential Information Participant may have in its possession or control.
Injunctive Relief. Participant acknowledges that any violation or threatened violation of this Section may cause irreparable injury to the other party, entitling the disclosing party to obtain injunctive relief in addition to all legal remedies.
Delivery and receipt of entries
DrivenData is not responsible for (a) late, lost, stolen, damaged, garbled, incomplete, incorrect or misdirected Entries or other communications, (b) errors, omissions, interruptions, deletions, defects, or delays in operations or transmission of information, in each case whether arising by way of technical or other failures or malfunctions of computer hardware, software, communications devices, or transmission lines, or (c) data corruption, theft, destruction, unauthorized access to or alteration of Submission materials, loss or otherwise. DrivenData is not responsible for electronic communications or emails which are undeliverable as a result of any form of active or passive filtering of any kind, or insufficient space in any email account to receive email messages. DrivenData disclaims any liability for damage to any computer system resulting from participation in, or accessing or downloading information in connection with, the Competition.
Reservation of rights
DrivenData, in its sole discretion, reserves the right to disqualify any person tampering with the entry process, the operation of the Web site, the competition process, or is otherwise in violation of the Competition Rules. DrivenData reserves the right at any time to disqualify a Submission from a Competition where, acting in good faith, it believes there are reasonable grounds to warrant disqualification. For example, DrivenData’s determination that the Submission does not provide the functionality described or required, or the Submission appears to be purposely designed to circumvent these Competition Rules or the spirit of the Competition would be grounds for disqualification. DrivenData reserves the right to cancel, terminate or modify the Competition if it is not capable of completion as planned for any reason, including infection by computer virus, bugs, tampering, unauthorized intervention or technical failures of any sort. If DrivenData determines (at any time and in their sole discretion) that any Participant is engaging in behavior that DrivenData deems obnoxious, inappropriate, threatening, illegal or that is intended to annoy, abuse, or harass any other person or entity, DrivenData reserves the right to disqualify such Participant (and Participant’s Team, if applicable).
General release
By entering the Competition, you release and discharge the Competition Sponsor and DrivenData from any liability whatsoever in connection with the Competition or with the acceptance, possession, use or misuse of any prize including, without limitation, legal claims, costs, injuries, losses or damages, demands or actions of any kind (including, without limitation: personal injuries; death; damage to, loss or destruction of property; rights of publicity or privacy; and defamation or portrayal in a false light). The Competition Sponsor and DrivenData will not be responsible for typographical, printing or other inadvertent errors in these Competition Rules or in other materials relating to the Competition. Additionally, you hereby agree to indemnify the Competition Sponsor and DrivenData from any and all losses, damages, costs, expenses, rights, claims, demands and actions (including attorney's fees and expenses for litigation and settlement), which may be brought against any one or more of them by anyone claiming to have suffered loss or damage as a result of your participation in the Competition.
Limitations of liability
By participating in the Competition, each Participant agrees that: (1) any and all disputes, claims, and causes of action arising out of or in connection with the Competition, or any prize awarded, shall be resolved individually without resort to any form of class action; (2) any claims, judgments and awards shall be limited to actual out-of-pocket costs incurred, including costs associated with entering the Competition, but in no event attorney's fees; and (3) under no circumstances will a Participant be permitted to obtain any award for, and each Participant hereby waives all rights to claim, punitive, incidental or consequential damages and any and all rights to have damages multiplied or otherwise increased and any other damages, other than damages for actual out-of-pocket expenses.
DrivenData, Inc., Competition Sponsor, and the employees, officers, directors, shareholders, agents, representatives, affiliates, subsidiaries, and advertising, promotional and legal advisors thereof (collectively, the “Released Parties”) are not responsible and shall not be liable for: (i) late, lost, delayed, damaged, misdirected, incomplete, illegible, or unintelligible entries; (ii) telephone, electronic, hardware, or software program, network, Internet, or computer malfunctions, failures, or difficulties; (iii) errors in transmission; (iv) any condition caused by events beyond the control of the Released Parties that may cause the Competition to be disrupted or corrupted; (v) any injuries, losses, or damages of any kind caused by a prize or resulting from acceptance, possession, or use of a prize, or from participation in the Competition (however (but only if required by law in your jurisdiction), this release and hold harmless commitment does not apply to cases of bodily injury or loss of life to the extent that any death or personal injury is caused by the negligence of DrivenData, Competition Sponsor, or other third parties, where liability to the injured party cannot be excluded by law); or (vi) any printing or typographical errors in any materials associated with the Competition.
Disclaimer of Warranties
You understand that we cannot and do not guarantee or warrant that the Data or other files available for downloading from the internet or the Competition Website will be free of viruses or other destructive code. You are responsible for implementing sufficient procedures and checkpoints to satisfy your particular requirements for anti-virus protection and accuracy of data input and output, and for maintaining a means external to our site for any reconstruction of any lost data. RELEASED PARTIES WILL NOT BE LIABLE FOR ANY LOSS OR DAMAGE CAUSED BY A DISTRIBUTED DENIAL-OF-SERVICE ATTACK, VIRUSES OR OTHER TECHNOLOGICALLY HARMFUL MATERIAL THAT MAY INFECT YOUR COMPUTER EQUIPMENT, COMPUTER PROGRAMS, DATA OR OTHER PROPRIETARY MATERIAL DUE TO YOUR USE OF THE WEBSITE OR ANY SERVICES OR ITEMS OBTAINED THROUGH THE WEBSITE OR TO YOUR DOWNLOADING OF ANY MATERIAL POSTED ON IT, OR ON ANY WEBSITE LINKED TO IT.
YOUR USE OF THE COMPETITION WEBSITE, ITS CONTENT AND ANY SERVICES OR ITEMS OBTAINED THROUGH THE WEBSITE AND RECEIPT OF ANY PRIZES IS AT YOUR OWN RISK. THE WEBSITE, ITS CONTENT AND ANY SERVICES OR ITEMS OBTAINED THROUGH THE WEBSITE ARE PROVIDED ON AN “AS IS” AND “AS AVAILABLE” BASIS, WITHOUT ANY WARRANTIES OF ANY KIND, EITHER EXPRESS OR IMPLIED, BEYOND ANY NON-EXCLUDABLE GUARANTEES (DEFINED BELOW). NEITHER THE RELEASED PARTIES NOR ANY PERSON ASSOCIATED WITH RELEASED PARTIES MAKES ANY WARRANTY OR REPRESENTATION WITH RESPECT TO THE COMPLETENESS, SECURITY, RELIABILITY, QUALITY, ACCURACY OR AVAILABILITY OF THE COMPETITION WEBSITE. WITHOUT LIMITING THE FOREGOING, NEITHER RELEASED PARTIES NOR ANYONE ASSOCIATED WITH THE RELEASED PARTIES REPRESENTS OR WARRANTS THAT THE COMPETITION WEBSITE, ITS CONTENT OR ANY SERVICES OR ITEMS OBTAINED THROUGH THE WEBSITE WILL BE ACCURATE, RELIABLE, ERROR-FREE OR UNINTERRUPTED, THAT DEFECTS WILL BE CORRECTED, THAT OUR SITE OR THE SERVER THAT MAKES IT AVAILABLE ARE FREE OF VIRUSES OR OTHER HARMFUL COMPONENTS OR THAT THE COMPETITION WEBSITE OR ANY SERVICES OR ITEMS OBTAINED THROUGH THE WEBSITE WILL OTHERWISE MEET YOUR NEEDS OR EXPECTATIONS.
NOTHING IN THESE COMPETITION RULES LIMITS, EXCLUDES, OR MODIFIES OR PURPORTS TO LIMIT, EXCLUDE, OR MODIFY ANY STATUTORY CONSUMER GUARANTEE OR ANY IMPLIED CONDITION OR WARRANTY, THE EXCLUSION OF WHICH FROM THESE COMPETITION RULES WOULD CONTRAVENE ANY STATUTE OR CAUSE ANY PART OF THESE TERMS TO BE VOID (“NON-EXCLUDABLE GUARANTEES”). SUBJECT TO THE LIMITATIONS IN THE PRECEDING SENTENCE AND TO THE MAXIMUM EXTENT PERMITTED BY ANY MANDATORY PROVISIONS OF APPLICABLE LAW, THE RELEASED PARTIES HEREBY DISCLAIM ALL WARRANTIES OF ANY KIND, WHETHER EXPRESS OR IMPLIED, STATUTORY OR OTHERWISE, INCLUDING BUT NOT LIMITED TO ANY WARRANTIES OF MERCHANTABILITY, NON-INFRINGEMENT AND FITNESS FOR PARTICULAR PURPOSE.
THE FOREGOING DOES NOT AFFECT ANY WARRANTIES WHICH CANNOT BE EXCLUDED OR LIMITED UNDER APPLICABLE LAW.
Governing Law and Jurisdiction
DrivenData shall be the sole interpreter of these Competition Rules.
All matters relating to the Competition Rules or the Competition and any dispute or claim arising therefrom or related thereto (in each case, including non-contractual disputes or claims), shall be governed by and construed in accordance with the internal laws of the Commonwealth of Massachusetts without giving effect to any choice or conflict of law provision or rule (whether of the Commonwealth of Massachusetts or any other jurisdiction).
Any legal suit, action or proceeding arising out of, or related to, the Competition or the Competition Website shall be instituted exclusively in the federal courts of the United States or the courts of the Commonwealth of Massachusetts although we retain the right to bring any suit, action or proceeding against you for breach of these Terms of Use in your country of residence or any other relevant country. You waive any and all objections to the exercise of jurisdiction over you by such courts and to venue in such courts.
The invalidity or unenforceability of any provision of these Competition Rules shall not affect the validity or enforceability of any other provision. In the event that any provision is determined to be invalid or otherwise unenforceable or illegal, these Competition Rules shall otherwise remain in effect and be construed in accordance with their terms as if the invalid or illegal provision was not contained herein.