Meta AI Video Similarity Challenge
Credit: BrentOzar
Participants in the Meta AI Video Similarity Challenge found creative ways to improve representations used for copy detection, as well as localization techniques that allow copied sections to be identified efficiently within longer videos.
Ed Pizzi, Meta AI Research Scientist and Video Similarity Challenge author
Why
The ability to identify and track content on social media platforms, called content tracing, is crucial to the experience of billions of users on these platforms. Previously, Meta AI and DrivenData hosted the Image Similarity Challenge in which participants developed state-of-the-art models capable of accurately detecting when an image was derived from a known image. The motivation for detecting copies and manipulations with videos is similar — enforcing copyright protections, identifying misinformation, and removing violent or objectionable content.
Manual content moderation has challenges scaling to meet the large volume of content on platforms like Instagram and Facebook, where tens of thousands of hours of video are uploaded each day. Accurate and performant algorithms are critical in flagging and removing inappropriate content. This competition allows you to test your skills in building a key part of that content tracing system, and in so doing contribute to making social media more trustworthy and safe for the people who use it.
The Solution
For this challenge, Meta AI compiled a new dataset composed of approximately 100,000 videos derived from the YFCC100M dataset. This dataset was divided into a training set, a Phase 1 test set, and a Phase 2 test set. Both the train and test sets are further divided into a set of ~40,000 reference videos, and a set of ~8,000 query videos that may or may not contain content derived from one or more videos in the reference set.
For the Descriptor Track, participants were tasked with generating useful vector embeddings for videos, up to one embedding per second of video, such that derived videos would receive high similarity scores to their corresponding reference video. For the Matching Track, participants were tasked with identifying the segments of a query video derived from corresponding segments of a reference video; Meta AI designed a segment-matching micro-average precision metric to measure performance on this Matching Track task.
Results
The winning solutions significantly improved on the baseline models provided by Meta AI. The top Descriptor Track solution improved on the baseline model by more than 40% (from micro-average precision of 0.60 to 0.87), and the top Matching Track solution improved on the baseline model by more than 105% (from micro-average precision of 0.44 to 0.92).
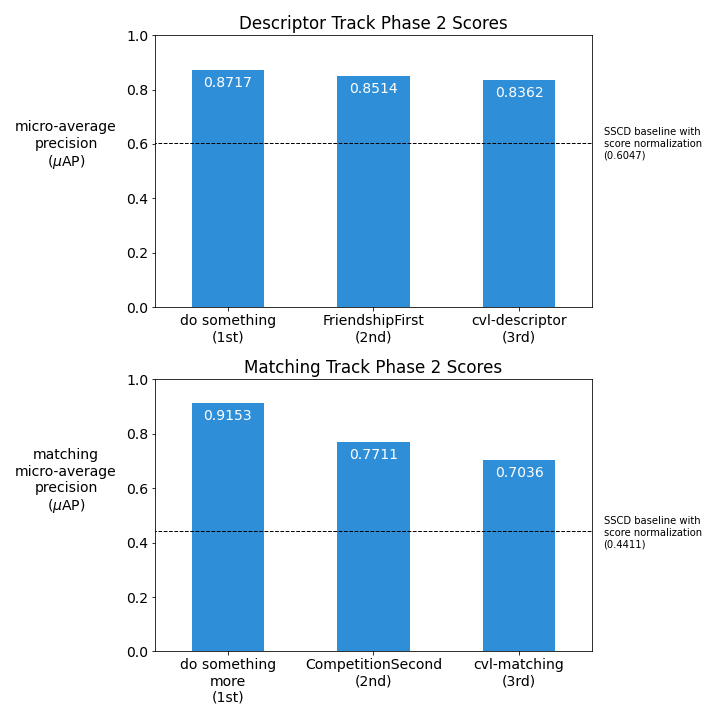
All the winners were invited to present their solutions at the Visual Copy Detection Workshop of the 2023 Conference on Computer Vision and Pattern Recognition (CVPR). Read more about the winning solutions in Meta AI's paper, our winner's blog post, and view the open-source solutions in the competition winner GitHub repository.
RESULTS ANNOUNCEMENT + MEET THE WINNERS
Phase 1

society
Descriptor Track | Phase 1
Help keep social media safe by identifying whether a video contains a manipulated clip from one or more videos in a reference set. #society

society
Matching Track | Phase 1
Help keep social media safe by identifying whether a video contains a manipulated clip from one or more videos in a reference set. #society
Phase 2

society
Descriptor Track | Phase 2
Help keep social media safe by identifying whether a video contains a manipulated clip from one or more videos in a reference set. #society

society
Matching Track | Phase 2
Help keep social media safe by identifying whether a video contains a manipulated clip from one or more videos in a reference set. #society
Open Arena

society
Descriptor Track | Open Arena
Help keep social media safe by identifying whether a video contains a manipulated clip from one or more videos in a reference set. #society

society
Matching Track | Open Arena
Help keep social media safe by identifying whether a video contains a manipulated clip from one or more videos in a reference set. #society